Khushi AI
In Rajasthan, India, 50,000 infants do not see their first birthday and 420,000 mothers go through high-risk deliveries - every year. We are developing AI models for early prediction of adverse maternal and neonatal care outcomes for 2.5M actively tracked mothers and infants across the state, so that 17,000 frontline nurses can prioritize their duelists and initiate early intervention.
In Rajasthan, India, 50,000 infants do not see their first birthday and 420,000 mothers go through high-risk deliveries - every year. While medical literature and local intuition can help guide which pregnant women and children are at higher risk of adverse outcomes, subtle nuances may go unnoticed leading to unexpected maternal and infant deaths. Rajasthan maintains records of over 80% of pregnancies (1.5 million) across the state, digitally, every year. However, data quality is cause for concern. Parameters for antenatal blood pressure, hemoglobin, and urine protein are often manipulated. Early and nuanced risk stratification of pregnant women therefore is hampered by poor quality inputs, despite the large volume of maternal and child health data being tracked.
Our solution is a series of machine-learning models to predict adverse maternal and child health outcomes. The solution comes in two-parts. First we work to solve the data-quality issue by training our models on gold-standard data (over 30,000 beneficiary records with 150 inputs each on health and social determinants from our rural laboratory in Udaipur, India). On this data set we have been able to fine-tune a set of over 30 data quality controls that give a composite index of the data being used in our prediction models. Now each input is weighted on the basis of it's reliability when used to train our prediction model.
Second, we design the prediction models using sophisticated neural networks which are fine-tuned over time as more data are provided. These models provide a probability of an adverse outcome for each pregnant woman and infant - allowing for prioritization of the health workers' due lists. Prioritized due-lists are conveniently shared along with action items to frontline nurses over WhatsApp groups. Our models which have shown 75% accuracy in predicting acute infant malnutrition are being now further refined in preparation to be applied to the State's repository of 2.5M beneficiaries.
Our solution primarily aims to empowers 17,000 frontline nurses (Auxiliary Nurse Midwives) who are responsible for caring for 2.5M pregnant women and infants living in rural Rajasthan, every year. Through timely delivery of prioritized due-lists, we hope to avert 2500 adverse infant outcomes by catalyzing timely intervention. The models structure is designed based on our understanding of behavior patterns over 4 years of closely working alongside 150 ANMs in rural Udaipur, Rajathan. The model outputs are equally catered to be delivered in a format that is both relevant and timely for the frontline nurse to take action.
- Expand access to high-quality, affordable care for women, new mothers, and newborns
Our solution seeks to expand access to high-quality, affordable maternal and newborn health care by helping frontline health workers identify which pregnant women and infants are at greatest risk of adverse outcomes, as suggested by our AI/ML models, which are locally refined over time. This prognostic tool may be used by frontline nurses to prioritize early intervention for these beneficiaries - advanced gynecological care referral, advanced planning for an institutional delivery, enhanced follow-up of maternal nutrition and adherence to micro-nutrient supplementation
- Pilot: An organization deploying a tested product, service, or business model in at least one community
- A new application of an existing technology
Precision public health approaches have yet to overcome a major hurdle of poor data quality from last mile settings. Khushi AI is working with partners at Google AI for Social Good and Singapore Management University to develop an automated data quality index for each data point reported by frontline nurses on maternal and child health data. This composite index considers 40 data quality rules, derived from deep understanding of frontline health worker usage patterns (e.g. manipulating all blood pressure values to show 120/80 even though the equipment is known to be faulty) from direct field experience and by applying statistical anomaly detectors to novel representations of the maternal and child health data set. This data quality index which precedes implementation of the machine learning model is the unique differentiator when compared to other models that (prematurely) assume the ground truth of the data being used to train the model.
The core technology behind Khushi AI involves a neural network algorithm, with a specialized filter for data quality, to predict maternal and child health outcomes from noisy and manipulated data entered from the field. This algorithm is applied to multiple distinct datasets including geolocation, social determinants and health factors. Health behavior outcomes and health outcomes are independently predicted with probabilistic scores. These scores are used to rank and feedback data to the frontline health worker via their mobile application for data collection and/or WhatsApp. Health workers are also incentivized to improve their data quality over time with social interventions. After recording the early interventions initiated, a separate algorithm is trained to minimize the likelihood of the previously established probability for the adverse maternal and child health outcome.
Our current model has been trained on a data set of 30,000 beneficiaries tracked through the Khushi Baby platform in rural Udaipur, India.
The following results describe the accuracy of the model in predicting moderate and severe acute malnutrition of infants, using data from the maternal and neonatal period:
Accuracy: 0.743 Precision: 0.759 Recall: 0.865 AUC: 0.795
Work is underway to refine the data quality layer using a combination of rules-based and data-based thresholds to define a field-validated data composite index used to weight each input.
- Artificial Intelligence / Machine Learning
- Big Data
- GIS and Geospatial Technology
- Software and Mobile Applications
We expect our data quality index and coupled social intervention to improve data quality to raise the standard of health data reporting from frontline health workers in the field over time.
By weighing each data input through our data quality index, and with sufficient diversity of inputs (including those from geographic, health worker, and beneficiary factors), we believe early signals can be identified for adverse maternal and child health outcomes, as evidenced by our early success with predicting acute malnutrition in infants.
We then hope to measure probability across a variety of health behavior outcomes and health outcomes through which a prioritized due list can be created.
Sharing these prioritized due lists in an automated manner with frontline nurses can allow them to prioritize follow-up to the top 10% of their 200-500 mother cohort. This early intervention is expected to lead to timely counseling, advanced gynecological screening, improved nutrition and medication adherence, and advanced preparation for delivery ultimately averting maternal and neonatal adverse events.
Outcome and intervention data are both collected and fed back into the model to improve its predictions over time. The model will benefit from the size of the big data set currently tracked by Rajasthan - 2.5M beneficiaries each with over 150 parameters.
- Women & Girls
- Pregnant Women
- Infants
- Rural
- Peri-Urban
- Urban
- Poor
- Low-Income
- Refugees & Internally Displaced Persons
- Minorities & Previously Excluded Populations
- 3. Good Health and Well-Being
- India
- India
Currently: 30,000 mothers and infants
This year: 2.5M mothers and infants (currently being refined for deployment with the state Department of Medical, Health, and Family Welfare)
Five years: 25M mothers and infants digitally tracked across India annually if experiment in Rajasthan is successful
To develop a model for precision public health care with actionable outputs for 200,000 frontline health workers across India taking care of 25M mothers and newborns every year.
1. The data quality itself presents a large barrier. Although historic data is abundantly available, high quality data is not.
2. Adoption of mobile platforms with higher degrees of systematically-ensured accountability checks are still in a nascent stage of adoption across Rajasthan and other early states in India. Mobile platform adoption will be critical to streamline high quality data on maternal and child health from frontline nurses
3. Funding support for large scale data quality validation, compute resources for the models, and investments required to ensure smartphone adoption across the frontline nursing cadre.
1. Our designation as the Nodal Technical Service Provider to the Rajasthan Department of Medical, Health, and Family Welfare gives us a tremendous opportunity to access an abundance of historic maternal child health data which can be used to inform models
2. Our partnership with Google AI and Singapore Management University is helping us develop sophisticated data quality validations that can be used to weigh individual records being passed from these big data sets
3. With the support of the DMHFW, we are deploying mobile applications to over 3500 frontline nurses in 5 Districts in the coming year who will collect data on 600,000 mothers and infants annually. Funding support has already been assured to support the technology costs (software, servers, internet plans) associated with this particular program.
- Nonprofit
38 full-time
4 part-time
Our team combines domain expertise in public health delivery with data science. Our 23 field and implementation managers have spent the last 3 years closely working with 150 frontline nurses in rural Udaipur to understand their patterns of data entry and service delivery at rural maternal and child health camps. Our research team includes a data scientist with prior experience in big data research for a major telecommunications company and an MPH graduate from Yale with concentration in epidemiology and global health, now in his final year at Harvard Medical School, with background in biostatistics and GIS.
We are working with researchers from Google AI for Social Good and a professor from Singapore Management University on development of the automated data quality index, used to weigh each data input for our machine learning models.
We also have a three year legal contract with the Department of Medical, Health, and Family Welfare, of the Government of Rajasthan to develop their integrated community health platform, Nirogi Rajasthan. Through this agreement we also are tasked with making the best use of their legacy platform for tracking maternal and child health, which tracks 2.5M beneficiaries annually. With this data set we will look to apply the learnings of our statistical models. Insights generated from these models will be shared through automated channels to department-employed health worker teams.
We are a non-profit in a 3-year, legal agreement with the Department of Medical, Health, and Family Welfare (DMHFW), for the Government of Rajasthan. Our contract ensures the DMHFW owns the solutions we build and implement. The DMHFW does not pay us for our services, but recognizes us explicitly as its Nodal Technical Service Partner for its flagship integrated health platform, Nirogi Rajasthan. Accordingly we use our affiliation to raise funds from donors and funders to support our internal operating costs, while we scale impact through IT-enabling the government's vast public health delivery system.
- Organizations (B2B)
We primarily raise funds from a combination of grants and partnerships with established funders. A small proportion of our funding comes through individual donors as well.
Our path to financial sustainability comes from continued and scaled delivery of our innovative platforms for demonstrable impact in the maternal and child health space, which leads to follow-on funding. Our R&D expands access to new grant and research based funding as well. Right now, we are in the process of raising a 1.5M ticket from private organizations to trigger 100% matching from the Bill and Melinda Gates Foundation, as a GAVI Infuse Pacesetter.
We are looking to partner with the best minds in big data management and AI for precision public health. Our previous experience working in the Early Childhood Track has shown us that the mix of solvers allow for solutions to grow through cross-pollination.
- Solution technology
- Talent recruitment
- Board members or advisors
We are looking to bring on technology experts to our board of advisors, hire top talent who is willing to join us in Jaipur, and further refine our approach to our machine learning solution.
We would be curious to see how data from IOT solutions, like that of former solver Neopenda, can be used to augment the longitudinal continuum of care driven machine learning predictions we are hoping to refine.
Our solution uses novel precision public health machine learning models to provide prioritized due lists of the highest risk mothers and neonates to female frontline nurses in rural India. In Rajasthan where we work, 420,000 mothers are at risk of complications during delivery every year. We hope that our algorithms can help catalyze targeted, early intervention to avert adverse maternal and neonatal outcomes.
Our solution uses novel precision public health machine learning models to provide prioritized due lists of the highest risk mothers and neonates to female frontline nurses in rural India. In Rajasthan where we work, 420,000 mothers are at risk of complications during delivery every year. We hope that our algorithms can help 17,000 frontline health nurses be prompted for targeted, early intervention to avert adverse maternal and neonatal outcomes.
Our solution uses novel precision public health machine learning models to provide prioritized due lists of the highest risk mothers and neonates to female frontline nurses in rural India. In Rajasthan where we work, 420,000 mothers are at risk of complications during delivery every year. We hope that our algorithms can help 17,000 frontline health nurses be prompted for targeted, early intervention to avert adverse maternal and neonatal outcomes.
Our solution uses novel precision public health machine learning models to provide prioritized due lists of the highest risk mothers and neonates to female frontline nurses in rural India. In Rajasthan where we work, 420,000 mothers are at risk of complications during delivery every year. We hope that our algorithms can help 17,000 frontline health nurses be prompted for targeted, early intervention to avert adverse maternal and neonatal outcomes.
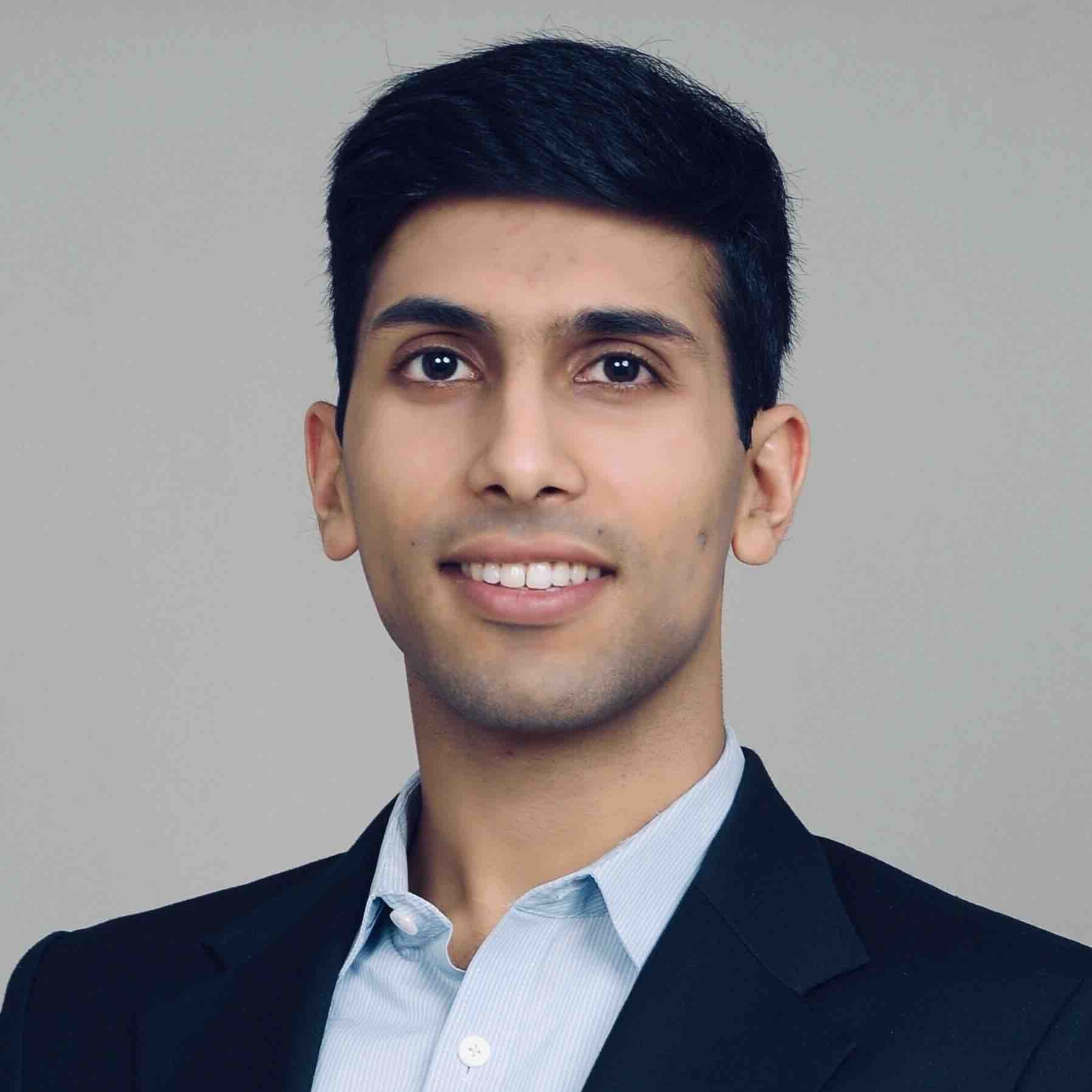
CEO
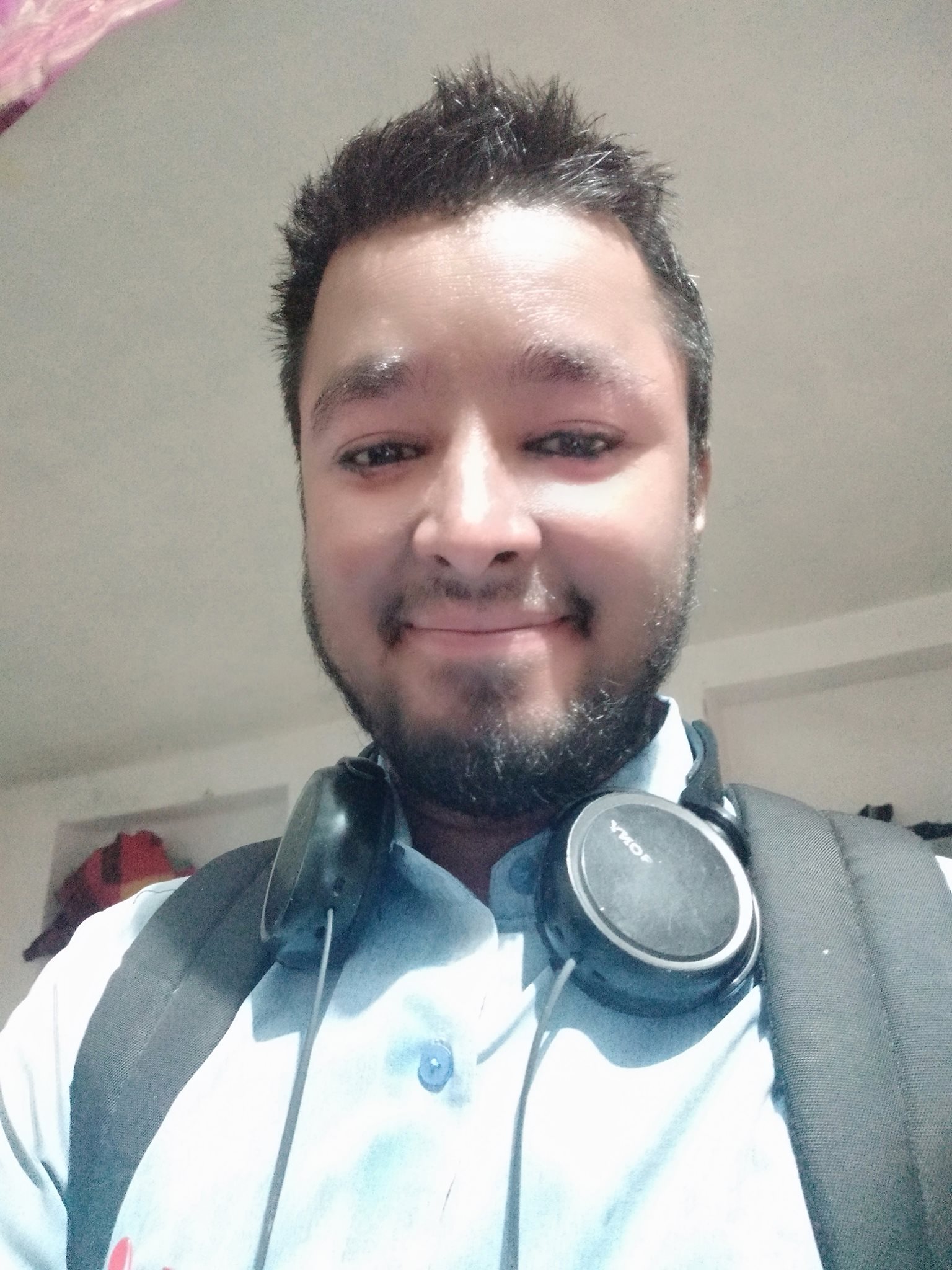
Chief Data Scientist, Technical Advisor, Academic Mentor
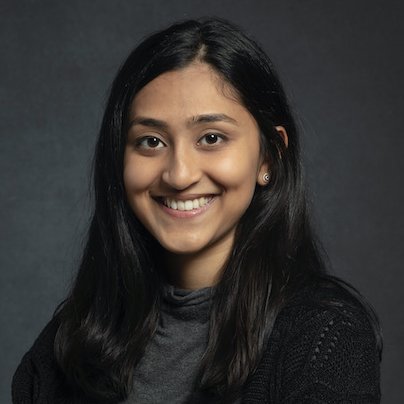
Strategy and Research Lead
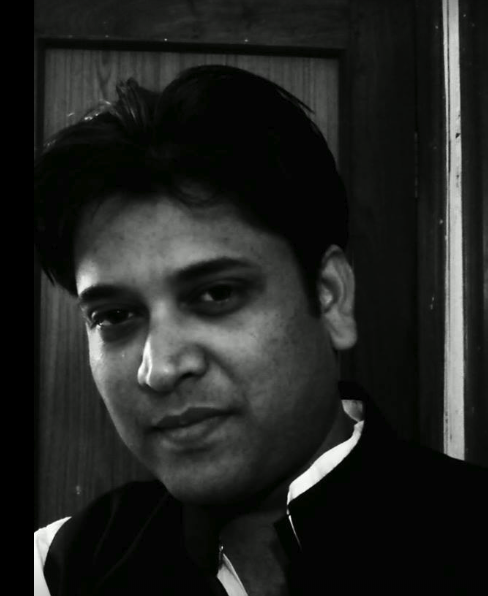
COO